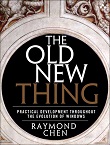
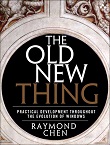
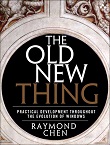
Adding state to the update notification pattern, part 6
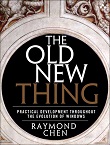
Adding state to the update notification pattern, part 5
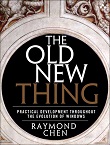
Adding state to the update notification pattern, part 4
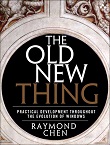
Adding state to the update notification pattern, part 3
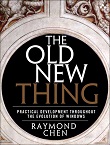
Adding state to the update notification pattern, part 2
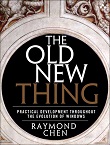
Adding state to the update notification pattern, part 1
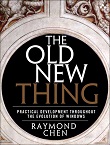
In search of the Ballmer Peak, and other results from SIGBOVIK 2024
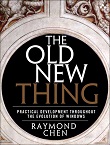
Dubious security vulnerability: Program allows its output to be exfiltrated
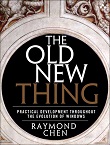