The Old New Thing
Practical development throughout the evolution of Windows.
Latest posts
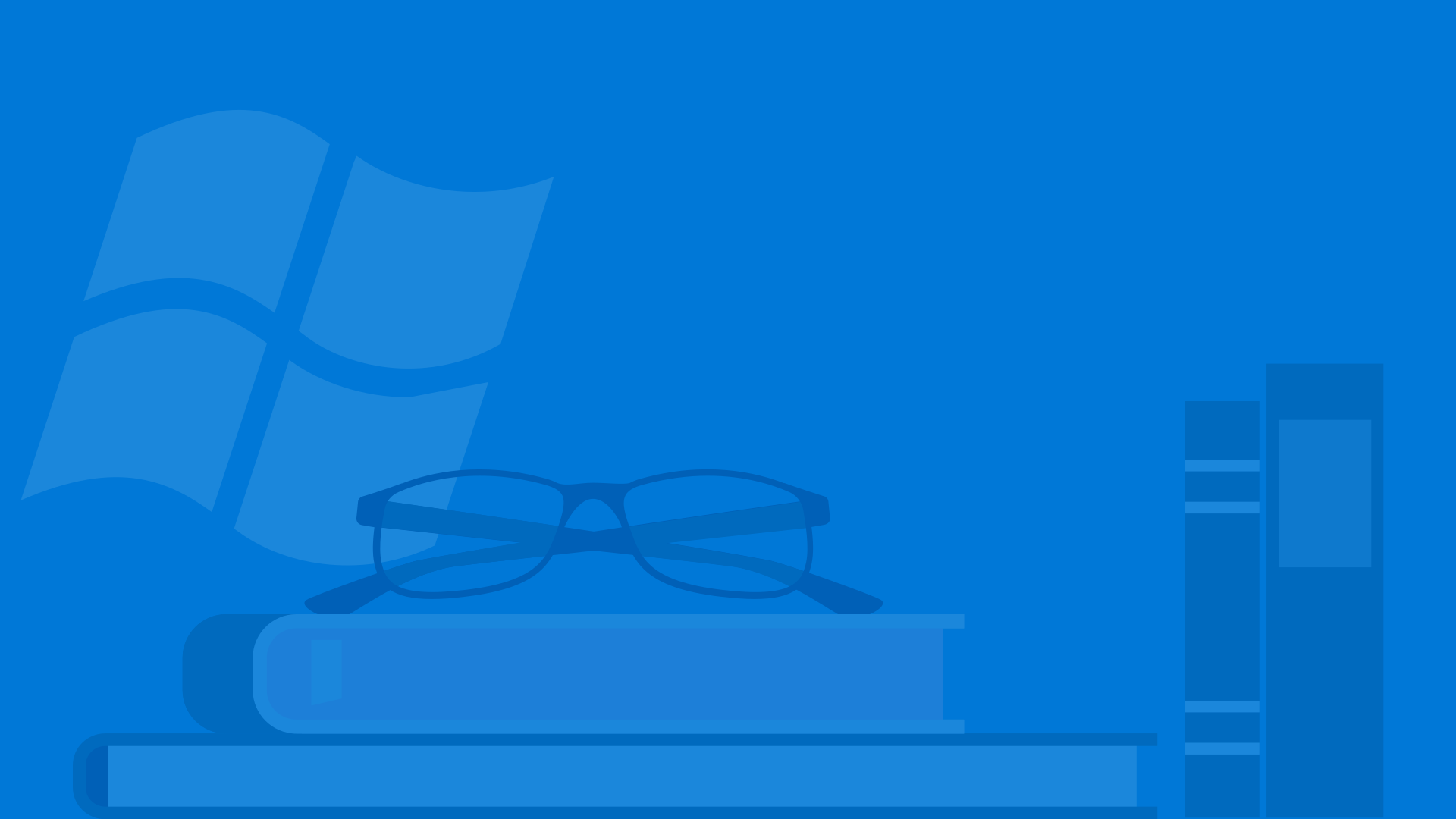
Jun 20, 2025
Post comments count
1
Post likes count
3
The case of the invalid handle error when a handle is closed while a thread is waiting on it
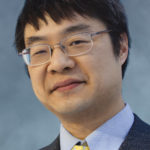
You are theorizing one race but experiencing another.
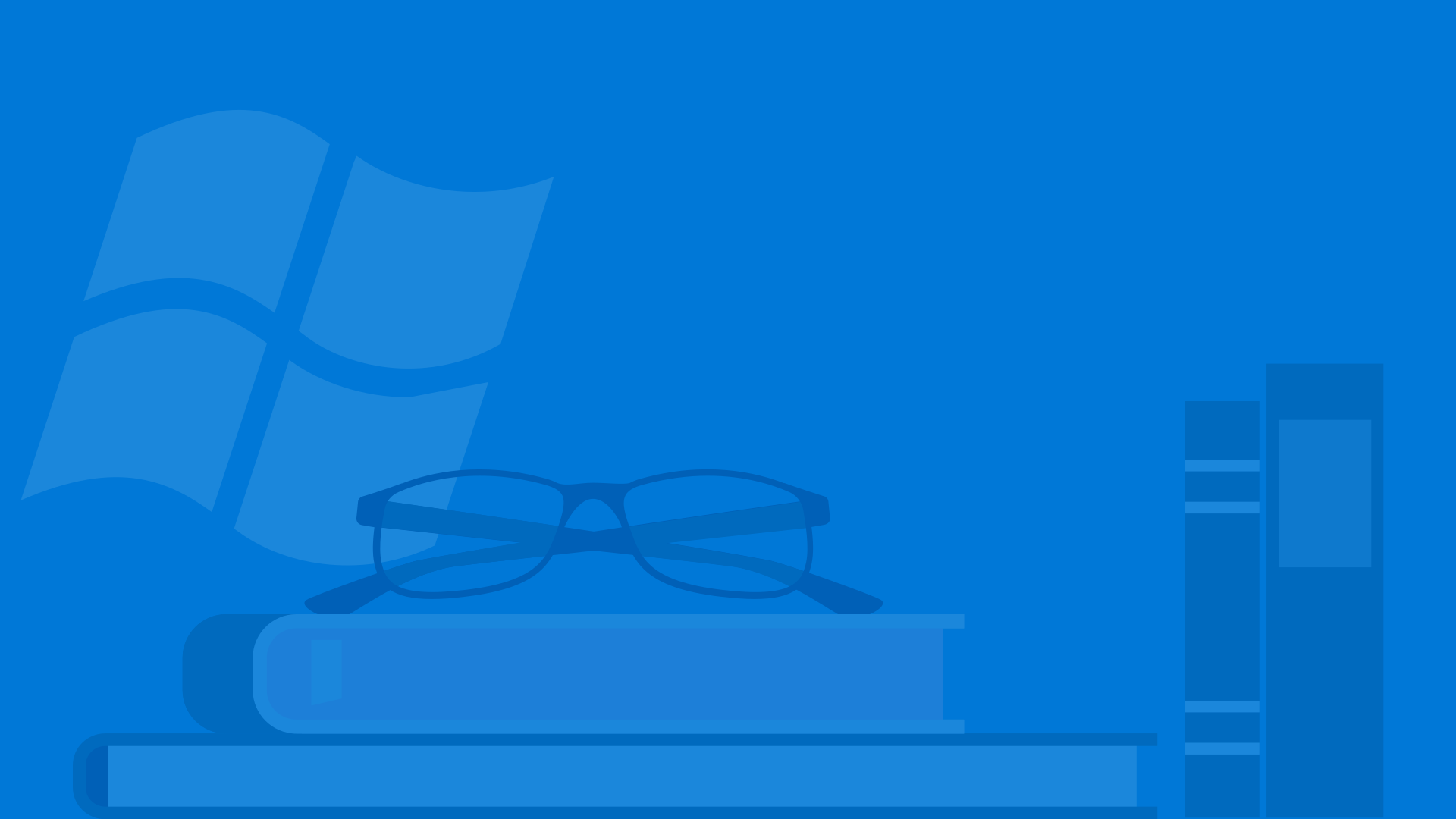
Jun 19, 2025
Post comments count
2
Post likes count
0
Learning to read C++ compiler errors: Ambiguous symbol errors after including a header file
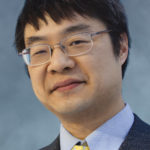
Finding out why multiple entities with the same name are visible.
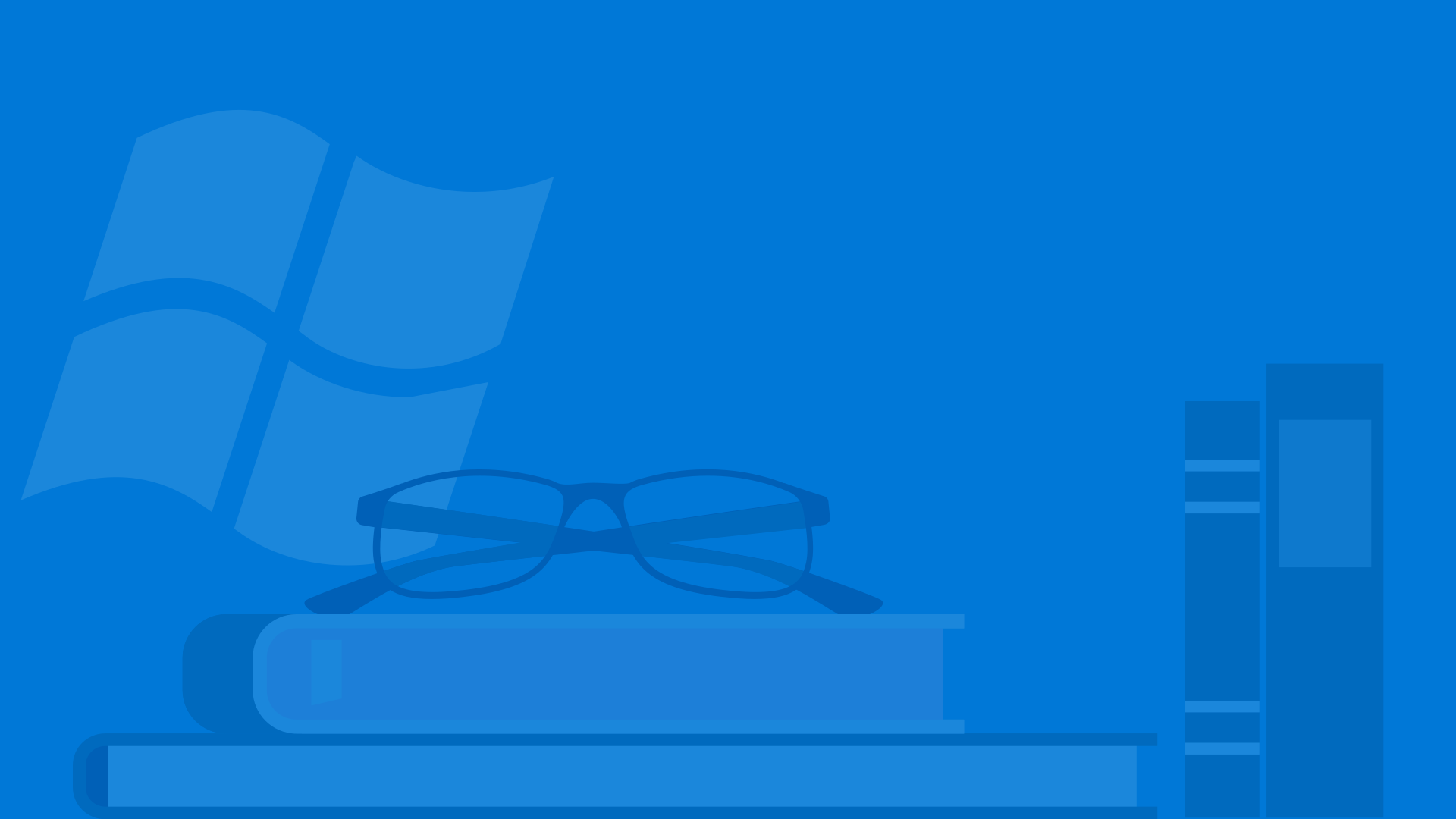
Jun 18, 2025
Post comments count
0
Post likes count
0
You have to tell Get-
and SetSecurityInfo
the object type, you can’t make it guess
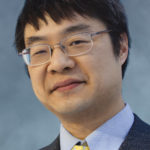
It needs to know which provider to give it to.
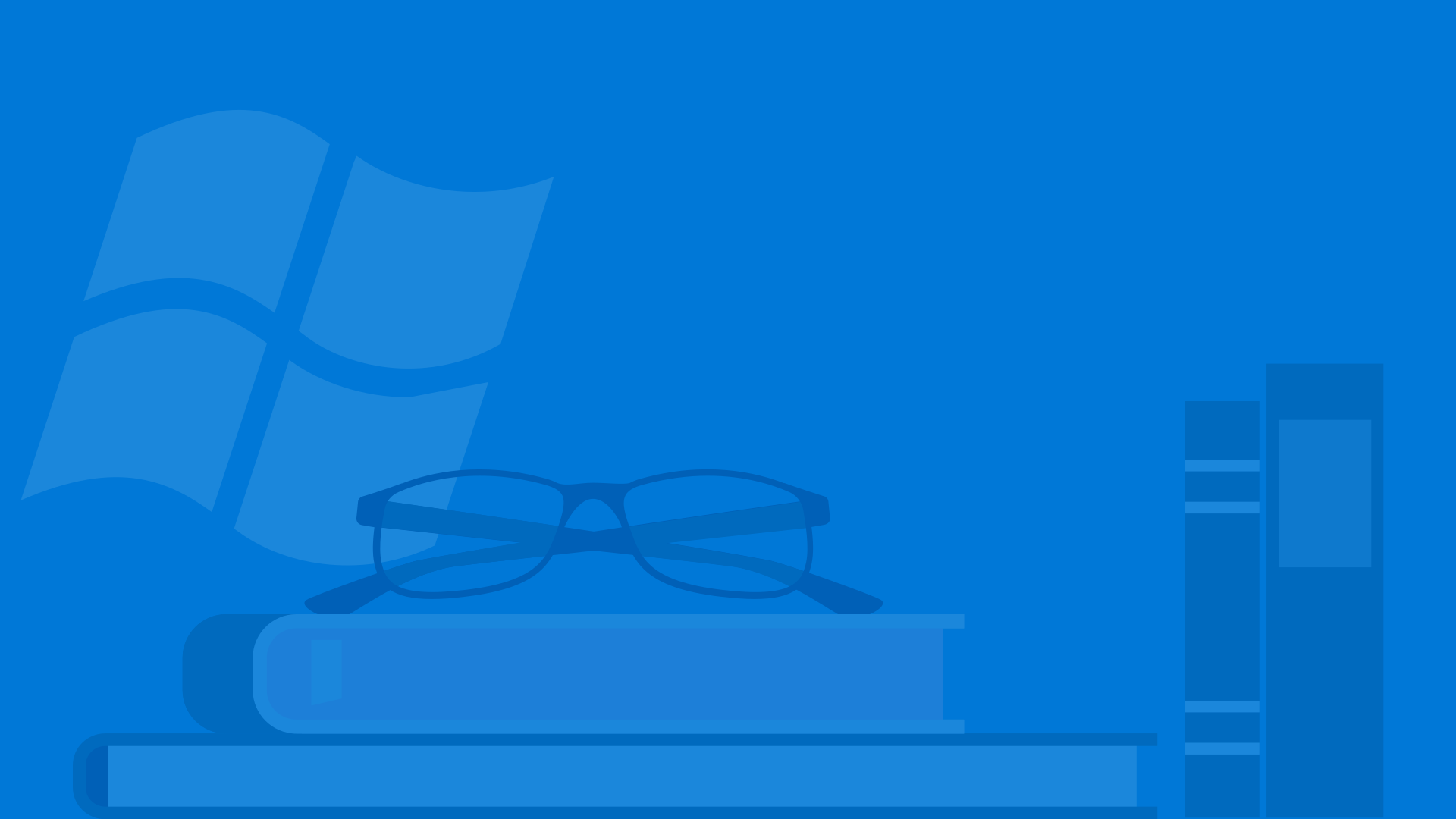
Jun 17, 2025
Post comments count
0
Post likes count
1
Funding the Egghead store shopping spree took a little extra legwork
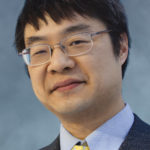
Just ask the boss.
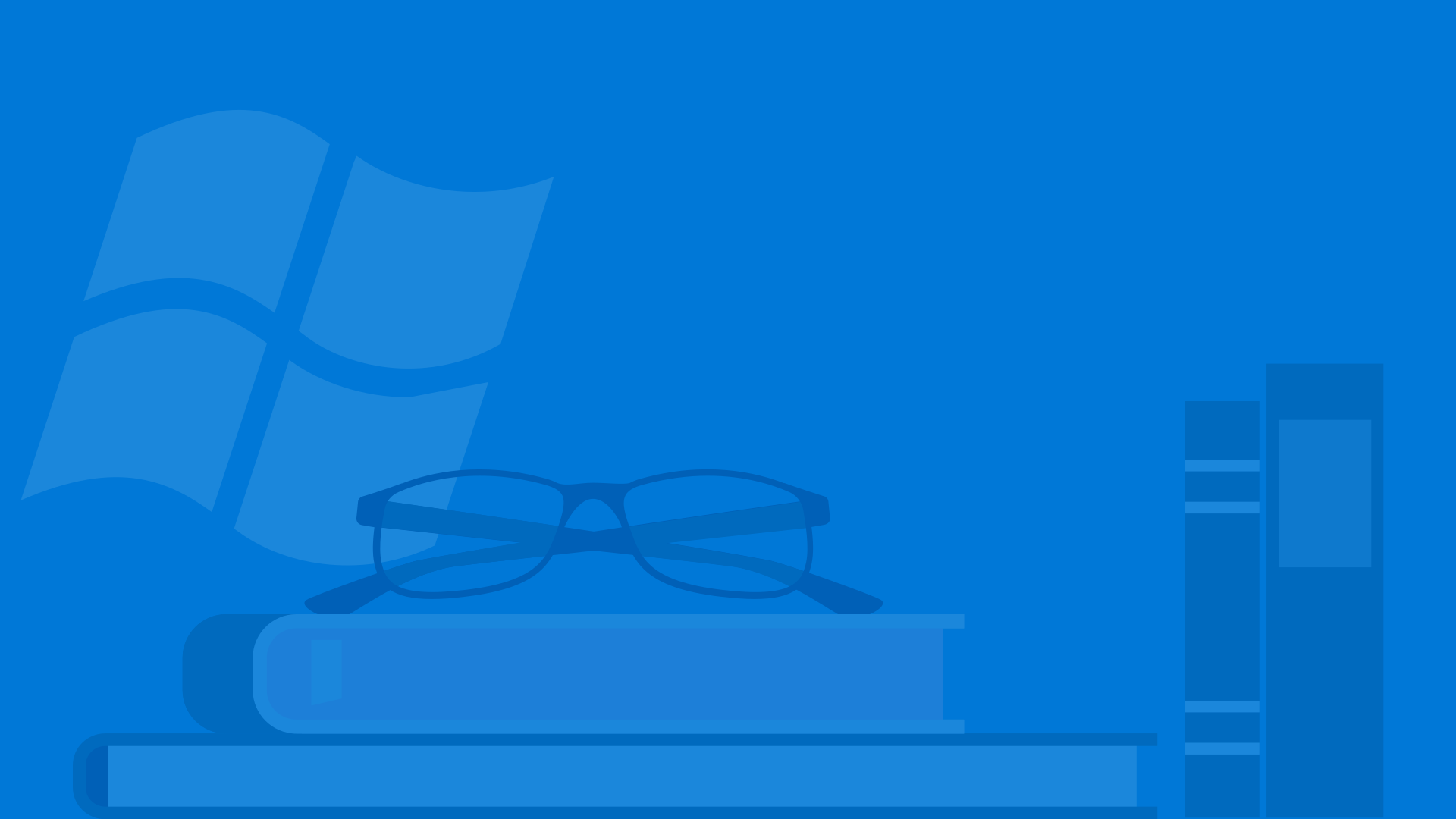
Jun 16, 2025
Post comments count
1
Post likes count
4
Writing a helper class for generating a particular category of C callback wrappers around C++ methods
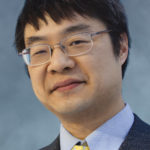
Another exercise in C++ template programming.
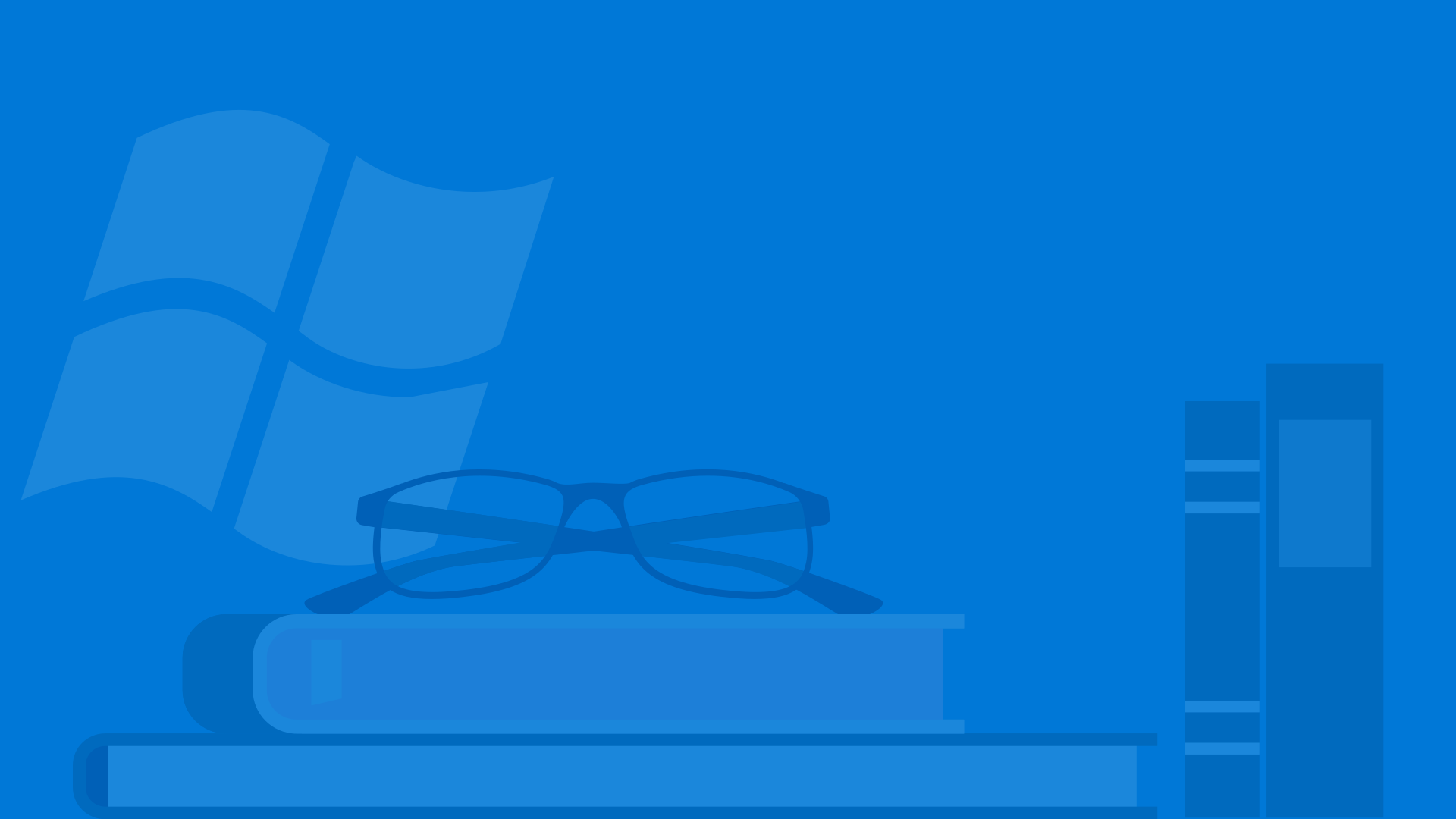
Jun 13, 2025
Post comments count
4
Post likes count
2
Thread pool threads are like preschool: Leave things the way you found them
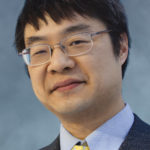
Your mother always told you to clean up behind yourself.
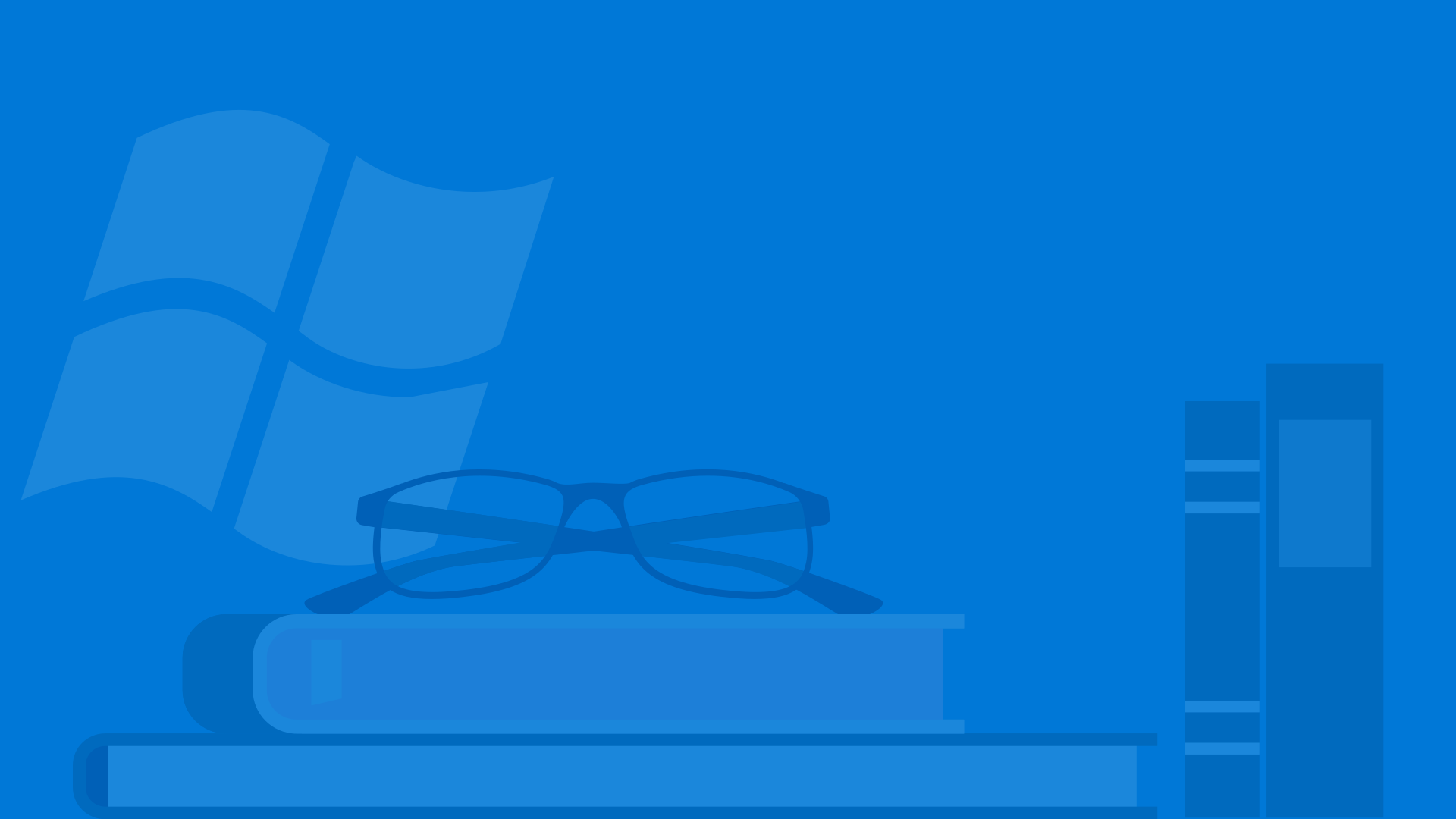
Jun 12, 2025
Post comments count
7
Post likes count
0
Why does Windows even have Interlocked
functions when we have std::atomic
?
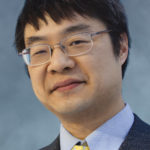
Well, you have to start somewhere.
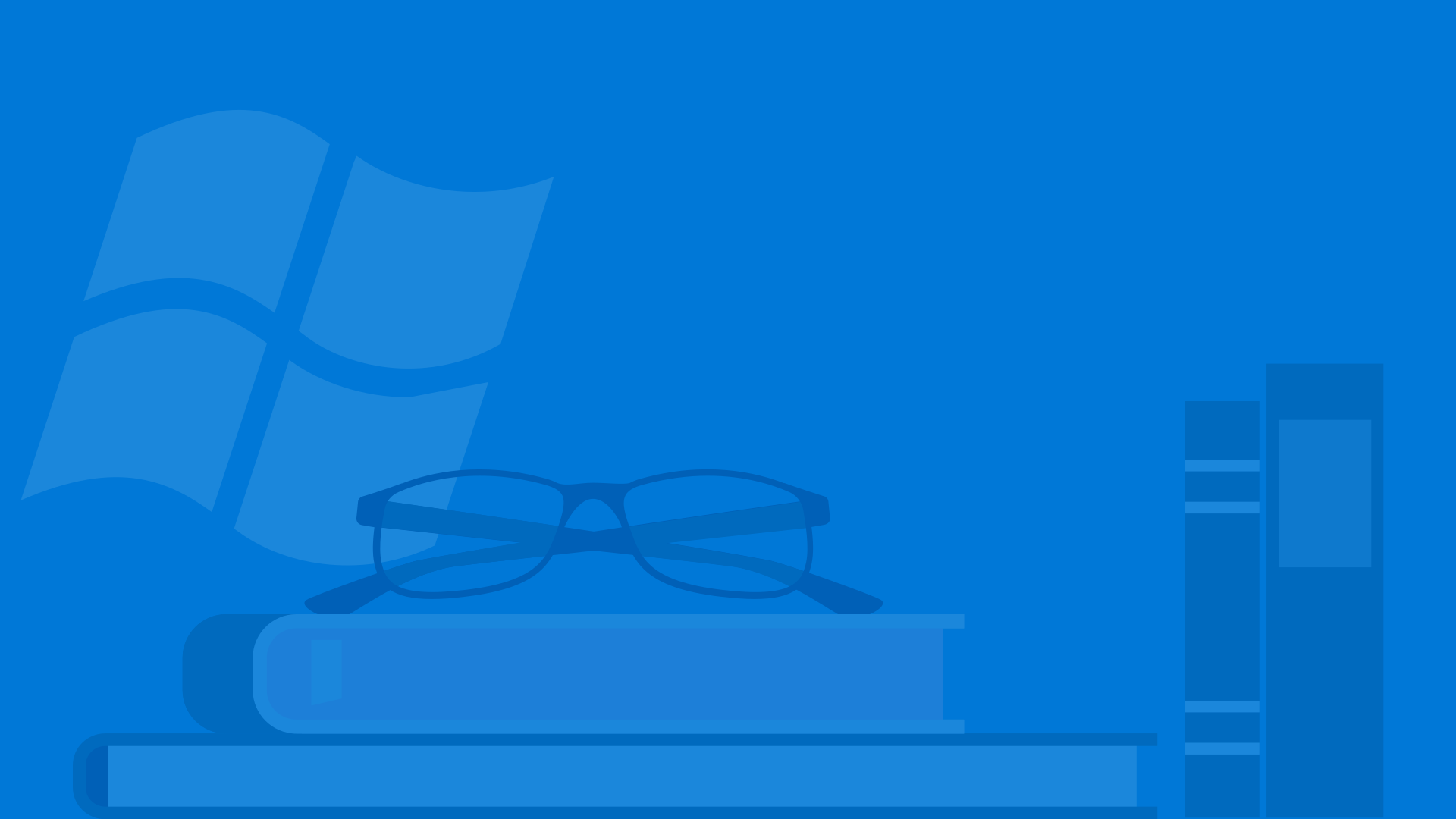
Jun 11, 2025
Post comments count
6
Post likes count
3
Removing the MAX_PATH
restriction on paths applies only to paths
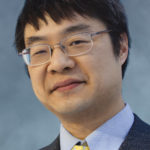
The individual file names still retain their existing limits.
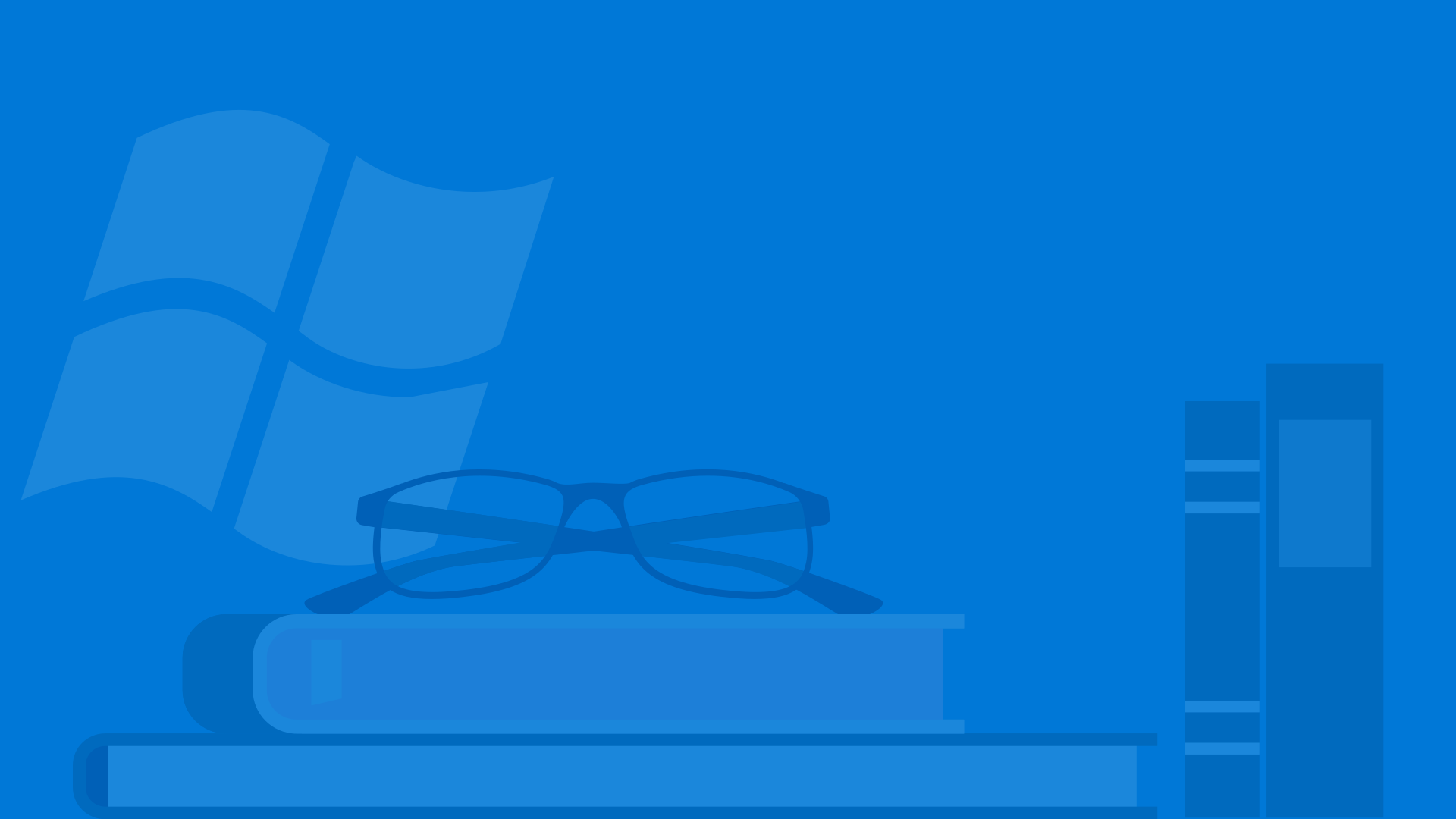
Jun 10, 2025
Post comments count
6
Post likes count
4
Application compatibility for Windows 95 crashed a cash register
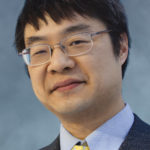
Exceeding all reasonable maximums.